Big data analytics is ushering in a new era of smarter supply chain management. By collecting and analyzing data from various sources, logistics and supply chain companies can enhance their operational efficiency and make smarter decisions about every aspect of supply chain management – from production and manufacturing, to procurement, sales and marketing, inventory management, distribution, and finance.
However, the path to seamless data-powered supply chain management can be a challenging one and organizations will need the right people, processes, technology, and partnerships to achieve data modernization and start generating value from their data.
In this blog, you’ll discover how organizations can leverage big data to build more resilient supply chains. We’ll also highlight three challenges that organizations face when it comes to operationalizing big data to enhance supply chain management. Finally, we’ll explore how modernizing data infrastructure and centralizing supply chain data in the cloud can help companies start leveraging big data analytics for smarter supply chain management.
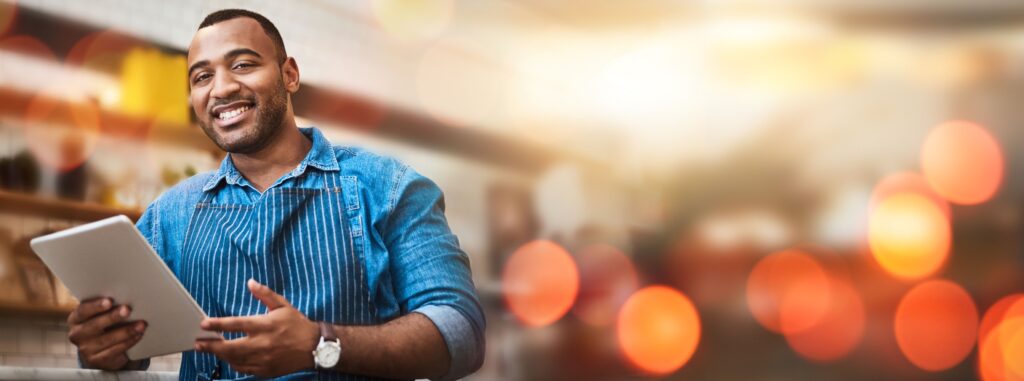
Six Use Cases for Smarter Supply Chain Management with Big Data
As supply chains grow more complex, companies are increasingly leveraging big data to boost productivity, reduce waste, and drive efficiency throughout the supply chain. With the right data infrastructure in place, companies can collect, aggregate, and analyze data from throughout their supply chains to enable a more efficient, data-driven approach.
Below, we highlight six of the best opportunities for logistics and supply chain companies to enhance operational efficiency with a data-driven approach.
1. Supply Chain Visibility
Supply chain visibility is the ability to easily and efficiently track products and materials as they move through the supply chain. By leveraging big data (e.g. real-time data from GPS or RFID-tagged shipments, shipment and delivery status data, supplier/partner data), companies can achieve real-time visibility of goods in the supply chain. This transparency allows for seamless coordination of supply chain activities and makes it easier to identify inefficiencies, respond to delays, or address bottlenecks.
2. Demand Forecasting
Demand forecasting leverages big data (e.g. historical sales data, consumer behavior data, marketing data, etc.) to predict future customer demand for specific goods or materials. Accurately anticipating customer demand for specific products allows companies to optimize their inventory levels and avoid overstock or stockout situations. Accurate demand forecasting is vital to maximizing profits, reducing storage costs, and ensuring customer satisfaction.
3. Supply Planning
Supply planning involves coordinating the procurement of materials, production schedules, and inventory management to meet the forecasted demand for products. By analyzing big data from throughout the supply chain (e.g. supplier performance data, inventory data, production capacity data, pricing and procurement cost data, etc.), companies can find ways to ensure timely availability of the materials they need while minimizing costs and avoiding delays.
4. Inventory Optimization
Inventory optimization relates closely to both demand forecasting and supply planning. By analyzing current and historical inventory data, historical sales data, pricing data, and supplier performance metrics like lead times and shipment quality, companies can order the right products in the right quantities at the right times to prevent stockouts that negatively impact customer satisfaction while avoiding overstock situations that waste valuable storage space.
5. Logistics and Route Optimization
Companies involved in supply chain management can analyze big data (e.g. historical delivery data, traffic and weather reports, delivery schedules and deadlines, customer location data, vehicle maintenance and fuel consumption data, etc.) to streamline their logistics operations by optimizing shipping and delivery routes.
Using big data for logistics and route optimization can result in lower fuel costs and faster delivery times for customers.
6. Financial Management
Efficient financial management and resource allocation are crucial components of effective supply chain management. By analyzing big data related to procurement and storage costs, revenue, profit margins, market pricing, and inventory, companies can improve financial management and planning while identifying the best opportunities to lower costs, increase revenue, and drive profits.
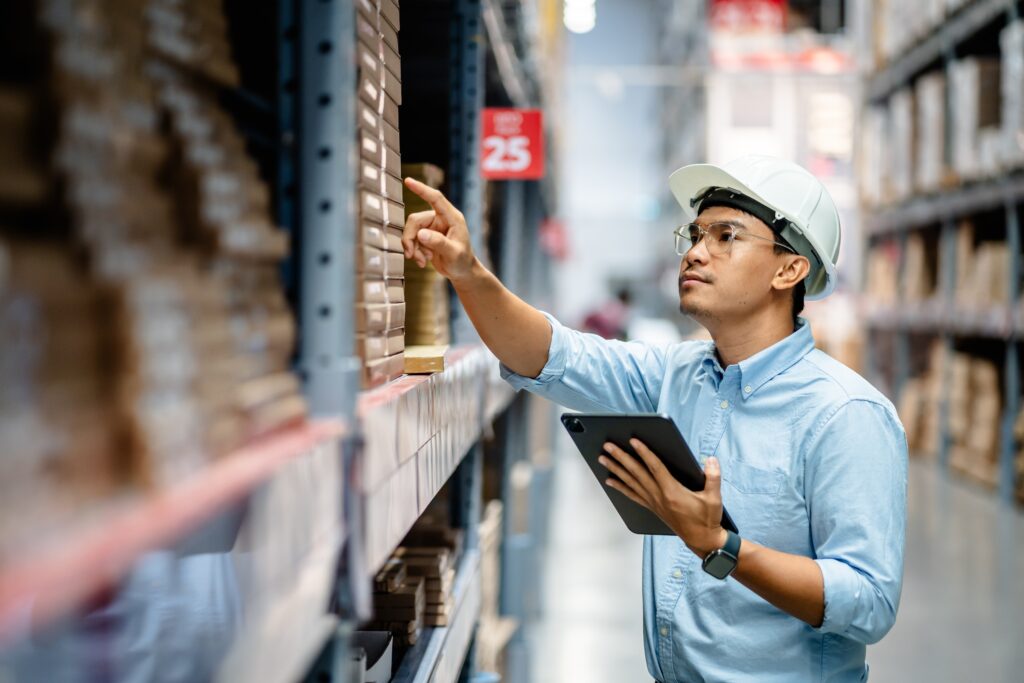
Three Big Data Challenges in Supply Chain Management
From demand forecasting and supply planning to inventory and logistics optimization, big data is creating valuable new opportunities for companies to manage their supply chain activities more efficiently.
But in order to realize the potential of big data analytics for supply chain optimization use cases, companies will need to invest in a data analytics platform like Snowflake that can be used to aggregate data from throughout the supply chain and analyze it to extract meaningful insights.
Companies will also need to overcome important challenges around data quality, sharing, and integration to effectively operationalize their supply chain data.
1. Data Sharing
Complex supply chains can involve hundreds of suppliers, manufacturers, logistics providers, and retail partners, each with their own IT systems, data formats, and processes. These differences can make it difficult for companies to efficiently share supply chain data with each other, resulting in slower time to insights, inaccurate forecasting, and poor collaboration.
2. Data Integration
To get the best insights, companies need to integrate data from many disparate sources into a single source of truth for supply chain analytics. Integrating data from many sources and formats into a unified system can be both complex and resource-intensive, requiring ongoing maintenance to keep data pipelines operating smoothly.
3. Data Quality and Governance
Generating useful supply chain insights requires effective data governance, as well as accurate, complete, and high-quality data. Inconsistent data entry practices, outdated information, poor data governance, slow or inefficient data collection processes, and lack of data standardization can all degrade the quality of data available to companies for supply chain analytics.
Logistics Companies Need Data Modernization to Enable Smarter Supply Chain Analytics
Before logistics companies can efficiently leverage their supply chain data for analytics use cases, they will need to modernize their data infrastructure and centralize enterprise data in the cloud. By transitioning from legacy on-prem systems to a primary cloud platform, supply chain companies can:
- Gain on-demand access to highly scalable, flexible, durable, accessible, and cost-effective public cloud infrastructure.
- Deploy modern cloud data platforms like Snowflake that make it easier to collect, store, and analyze data at scale.
- Increase the visibility, accessibility, and availability of supply chain data to support analytics use cases.
- Take advantage of emerging AI technologies to enable AI-powered route optimization, inventory management, and supply planning.
Reports that 89% of companies have adopted a multi-cloud approach, or that 73% of companies have adopted a hybrid cloud architecture can create the perception that most companies have already achieved data modernization. However, research from 2023 found that just 29% of organizations had centralized their data in the cloud and the majority were still storing data in on-prem legacy systems where it was difficult to access and utilize.
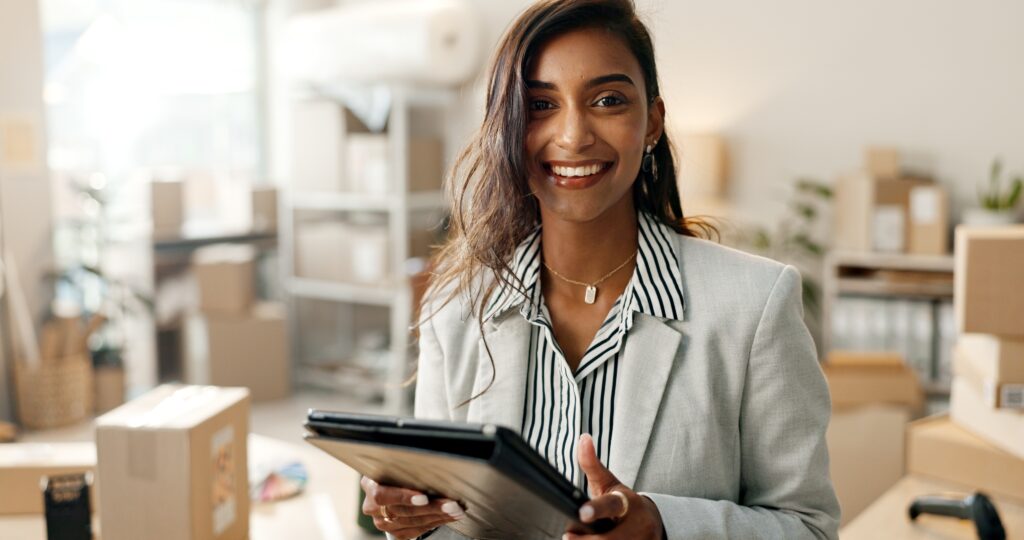
Building More Resilient, Smarter Supply Chains with Hakkoda and Snowflake
Supply chain companies are recognizing the huge potential of big data analytics when it comes to supply chain management.
Our 2024 State of Data Report revealed that 46% of logistics and supply chain companies are planning to centralize data on a primary cloud platform this year, but we also learned that nearly half of logistics businesses failed to achieve their data goals last year.
Without the right support, there’s a real risk that logistics companies could again miss their targets when it comes to modernizing data infrastructure and implementing supply chain analytics.
Hakkoda is the ideal data modernization partner for your supply chain or logistics company. Our experienced team will help you modernize your data infrastructure and centralize your data in the cloud by adopting the Snowflake AI Data Cloud, the world’s leading cloud-based data platform.
With secure, real-time data sharing across organizations, support for multiple data formats, and built-in data quality monitoring, migrating to Snowflake makes it easy to overcome data sharing, integration, and quality challenges and get the most value from your data.
Hakkoda’s team of 100% SnowPro certified engineers, architects, data scientists, and analysts can help you design the right data architecture and strategy for your needs, enable data sharing with other organizations in your supply chain, implement data governance policies to manage data access and quality, configure cost-effective data storage in Snowflake, and start generating high-quality insights from your supply chain data.
If you’re excited by the prospect of modernizing your data infrastructure and centralizing data in the cloud to enable supply chain analytics use cases, let’s have a conversation about streamlining your data journey with Hakkoda.
The post Leveraging Big Data for Smarter Supply Chain Management appeared first on Hakkoda.