With mergers and acquisitions comes rapid growth and exciting new possibilities, but when it comes to data integration tools, these moments of expansion can quickly become logistical nightmares. The crucial challenge is to standardize and synthesize disparate data quickly and effectively, creating a sustainable workflow and avoiding disruptions to day-to-day operations.
To try and adapt quickly, some organizations will aggregate the data into C-level dashboards, but the expediency of this solution comes at a cost. In the short term, the data is integrated in a functional manner, but valuable insights are lost at the granular level, which limits an organization’s ability to analyze the performance of the acquisition over time.
Another solution is to use the data vault as an engineering pattern, which can also allow for quick data integration. Originally, the data vault methodology was developed as a data modeling methodology that implemented the enterprise data warehouse. However, the data vault has evolved to become a data engineering pattern for the enterprise data pipeline, working in combination with modern tools like the Snowflake AI Data Cloud and Coalesce to boost productivity while facilitating smoother, more efficient organizational change.
In this blog, we’ll take a look at how you can use the data vault as an engineering platform to create a data integration tool with the power to drive data efficiency and synergy, especially in the aftermath of mergers and acquisitions.
Minimizing Risk and Simplifying Processes Through the Data Vault Engineering Pattern
Mergers and acquisitions offer a use case that demonstrates just how powerful the data vault can be, as a data engineering pattern. Let’s first consider the data pipeline for the enterprise. To illustrate this, we will focus on the customer as a key business concept. In this example, customer data will be coming from an enterprise master ERP data source like SAP. With the data vault being used as an engineering pattern, the data was engineered to the information mart.
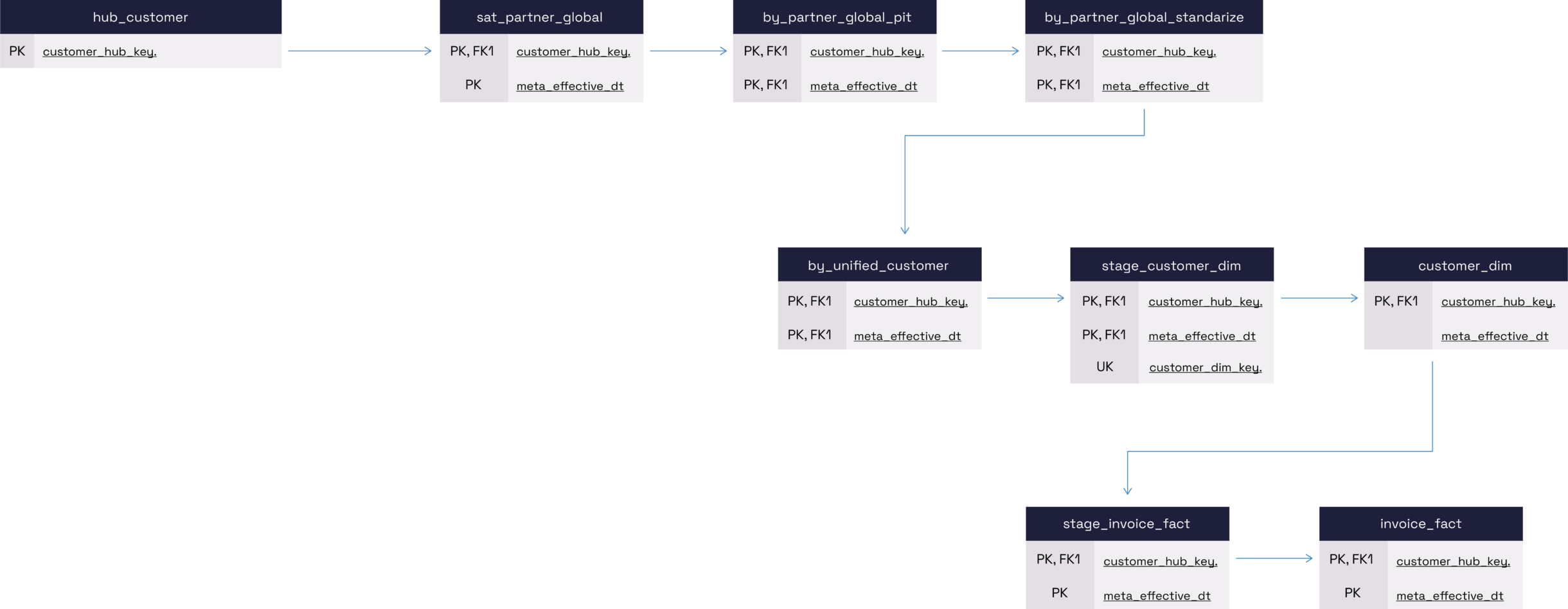
In this example, the data engineer used the data vault as an engineering pattern to build the customer data product flow. Figure 1 illustrates the data products produced as by-products along with the terminal objects of the dimension and fact table. The intermediate stage tables to build up the data are not shown, for the sake of simplicity and clarity.
Now let’s assume the acquisition of the Acme Company has been introduced. The company had their own ERP system, which was used to maintain their customer master data. The integration of the data follows the same data vault engineering pattern.
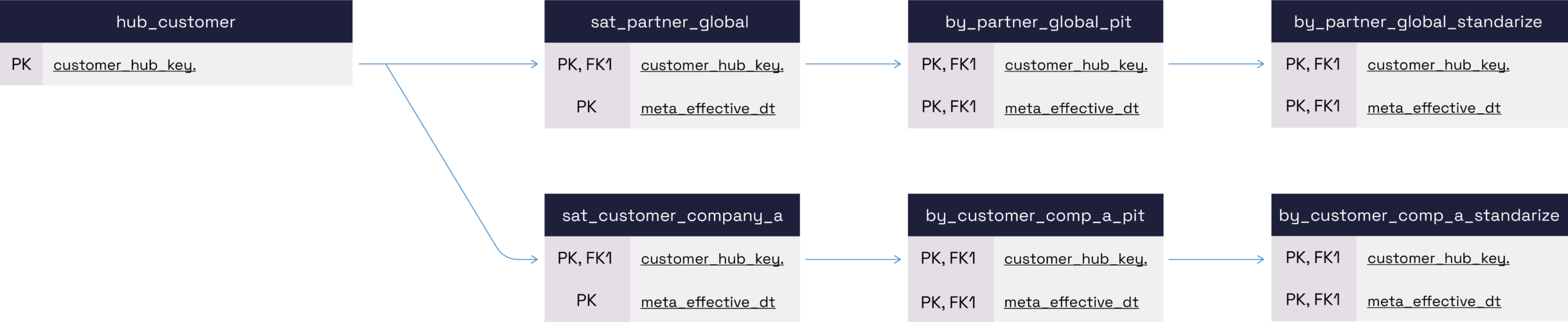
Any engineer can add new data to the enterprise data pipeline by following the data vault engineering pattern, without any specialized knowledge. They would simply need to follow the prescribed system.
One of the advantages of this model has to do with integrating the new customer data into a unified customer view. In figure 1, the unified customer data product feeds the downstream customer common dimension. The inclusion of the Acme data occurs in a single data pipeline, the unified customer data product.
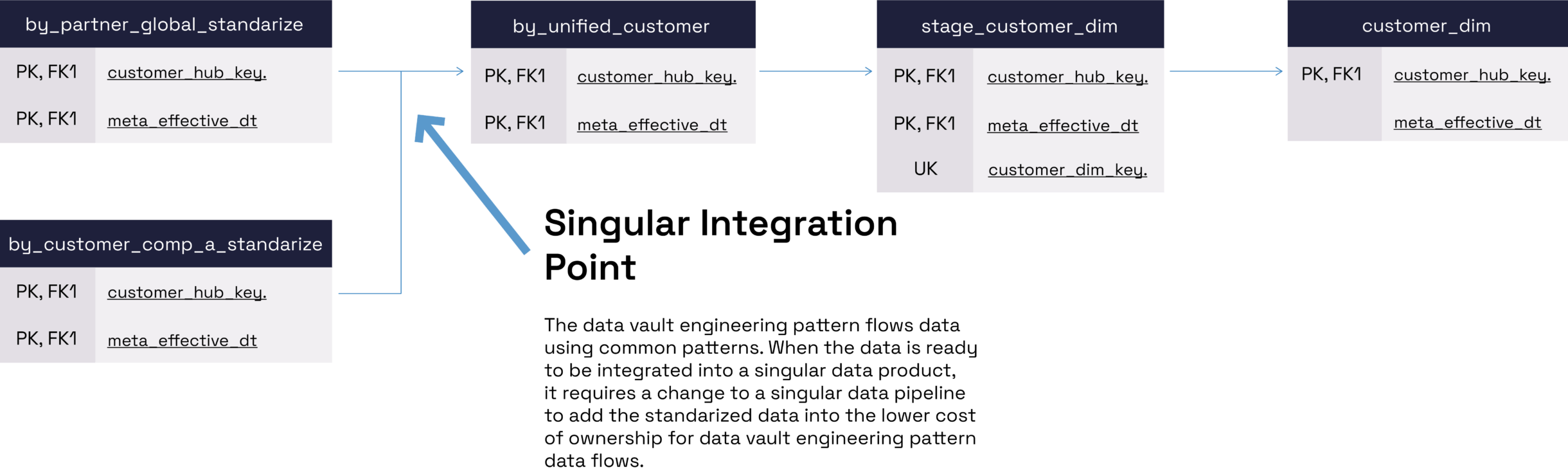
Deploying the data vault as an engineering pattern reduces the total cost of ownership of enterprise data platforms by simplifying integration points. By isolating change to a single data pipeline, organizations mitigate their data integration risks. The data vault engineering pattern makes it easier to both add the new data and maintain the data pipelines.
A Data Integration Tool that Preserves Data Pipelines While Driving Efficiency
Another benefit is the fact that modifying the data to integrate standardized data does not impact the schema definition of bv_unified_customer. In this way, data consumers who rely on this data product benefit from the new data without altering their pipelines.
By using the data vault pattern, data teams can continue to integrate data over time with minimal impact on existing pipelines. This approach also makes it possible for centralized data teams to quickly integrate M&A data to unlock performance analytics that have historically been locked away in the siloed system.

When this pattern is powered with modern data tools such as Snowflake and Coalesce, the data team’s velocity and efficiency naturally increases, thanks to the low-code automation which implements the patterns through templates.
With this combination of tools, the data engineer doesn’t need to be a data modeling specialist to deploy the data vault engineering pattern. There’s no need for advanced skills or special knowledge, as the pattern is well-documented in such a way that engineers of any skill levels can understand.
Optimize Your Data Integration Journey with Hakkōda
Data integration can be such a pivotal process for organizations experiencing sudden growth—that’s why it’s essential to make you have the tools and expertise you need to make the process smooth and successful.
At Hakkoda, our Snowflake experts are here to help you harness the most powerful data integration tools and strategies available in order to build the solutions that allow you to get the most out of your data now.
If your organization is undergoing sudden growth, let’s talk today about how Hakkoda can help you optimize your data integration journey.
The post Data Vault as Engineering Pattern: The Ultimate Data Integration Tool for Mergers & Acquisitions appeared first on Hakkoda.